May 14, 2024
Value preparation over generation
Currently, there is significant emphasis on using Generative AI (GenAI) to create more content. Beyond the well-documented legal, ethical, and governance risks associated with GenAI, prioritizing value preparation over generation serves as an overarching mitigation strategy for delivering future-ready outputs.
Being pragmatic and practical can be frustrating, and it may seem to constrain innovation during a period of massive, rapid technological disruption. However, having the right data to train your models to achieve optimal outputs is strategically your best next move. This isn’t merely a choice between low risk or high innovation, but rather a balance where considered risk can unlock innovation. You need a combination of both risk and innovation to make progress.
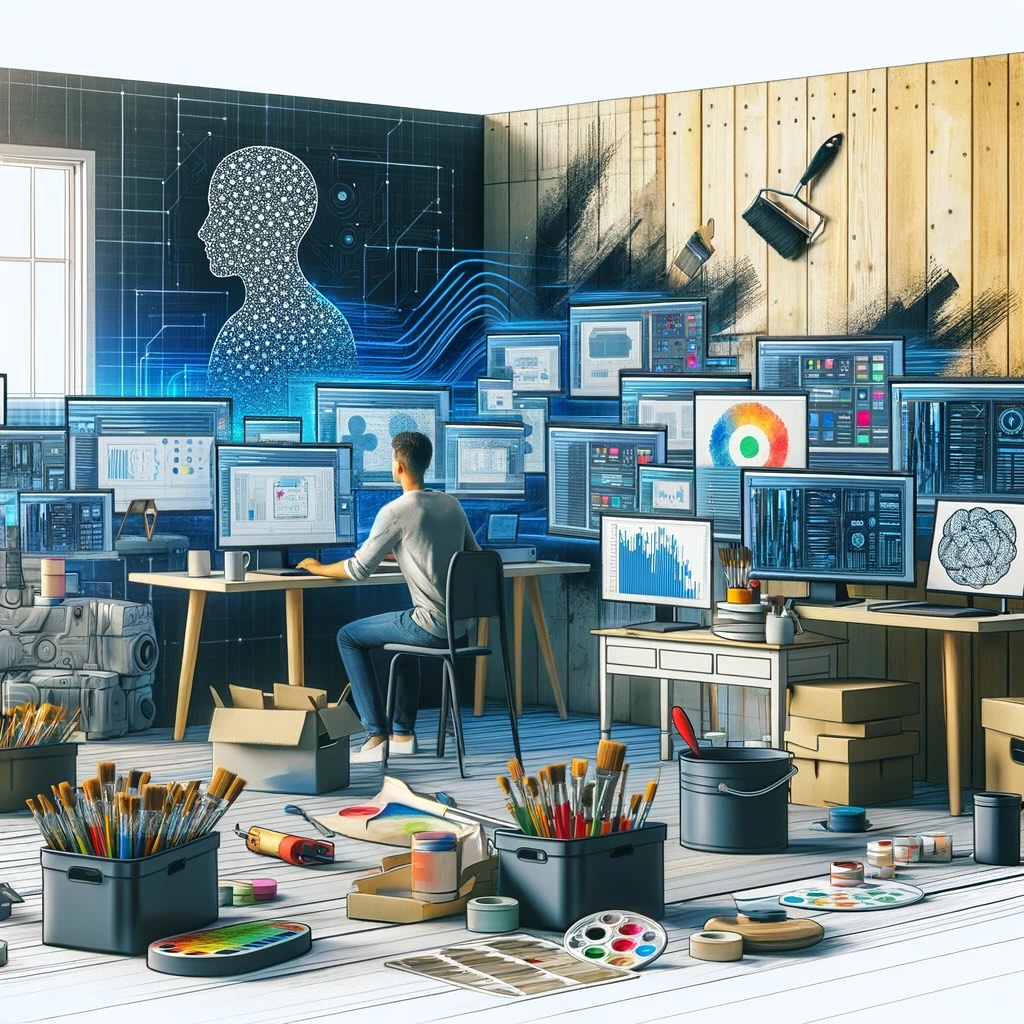
Value Preparation over Generation
In the rush to generate, many are shortcutting preparation. This approach may yield short-term gains but fuels long-term pain. When decorating a room, for example, it’s about 95% preparation and 5% painting. Stripping back to the plaster, smoothing out cracks, sanding down the woodwork, and cleaning debris are time-consuming but necessary preparation tasks. The quality of the final coat depends heavily on the quality of these preparatory steps.
GenAI is no different. The various hoops you must jump through to prepare data for training are critical to ensuring the accuracy and quality of downstream generative outputs. It’s the small things that count. For instance, we discovered that when preparing assets to train a brand-specific custom model, centering the images within the digital asset was crucial. The more training images required, the more manual effort needed during the preparation phase. This quickly becomes a hurdle that automation can help overcome—and we are making strides in this area. However, this is just one of many tasks being added to a growing pipeline of preparation tasks.
In Summary
The importance of data preparation in training AI models is a burgeoning area of research. Invest time to take the long way around. Only then can you creatively, iteratively, and incrementally shorten the distance between your inputs and desired outputs.